Using Multiple AI Assistants: A Pioneer's Perspective
Dust maximizes the potential of LLMs like GPT-4o, Claude 3, Gemini 1.5 Pro, and Mistral Large through innovative product layers. The real magic happens at the interface between people, company data, and top LLMs. Dust's approach enables businesses to leverage AI for transformative results fully.
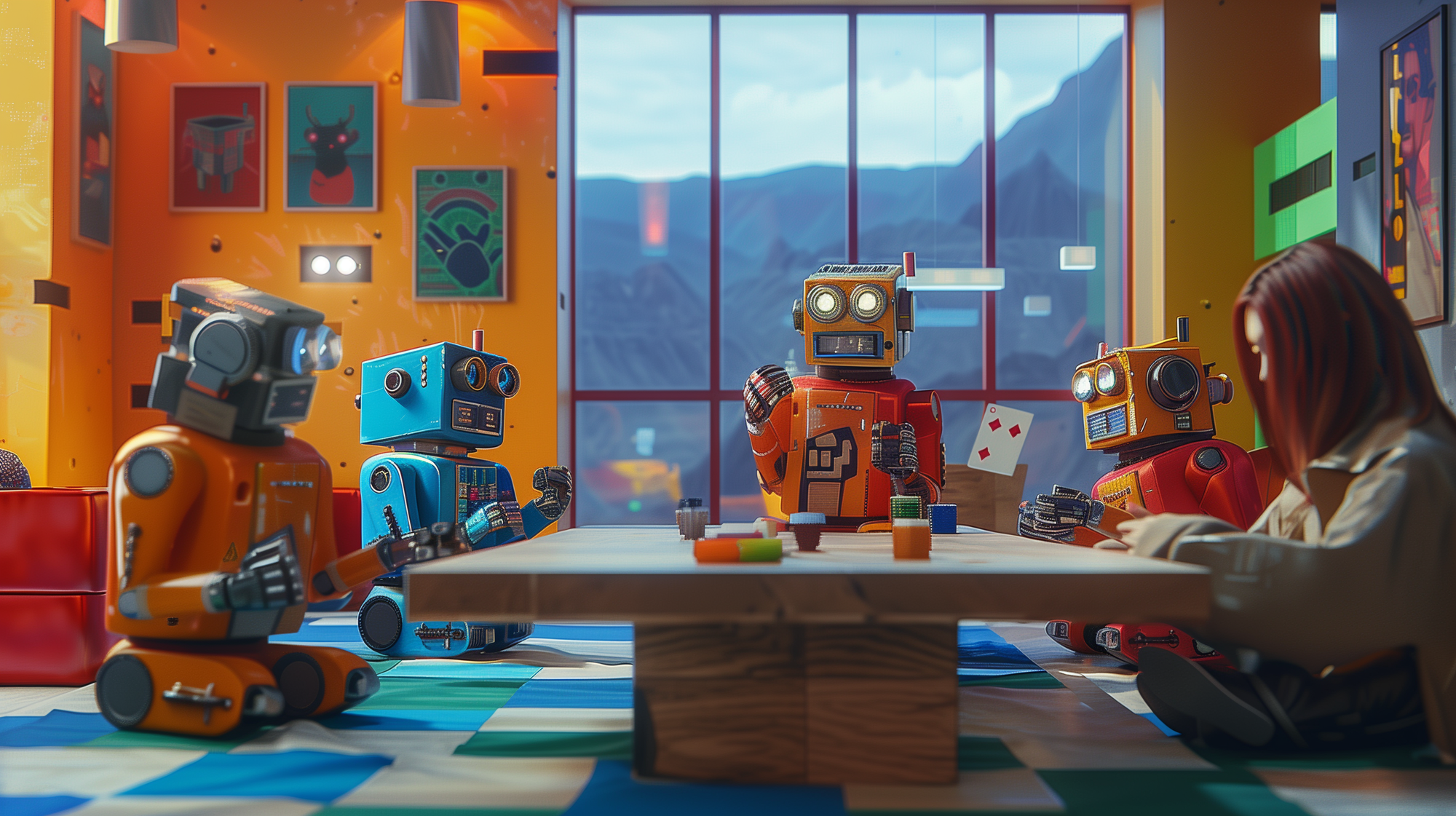
At Dust, we've always believed that building the right product layer is the path to unlocking the full potential of large language models (LLMs). While training frontier models get a lot of attention, we think the real magic happens at the interface between people, company data, and best-in-class LLMs: GPT-4o, Claude 3 family, Gemini 1.5 pro, and Mistral Large.
That's why we launched our multi-assistants feature in September 2023. This feature allows any company employee to create custom AI assistants for their specific needs and share them with teammates.
While Quora announced a similar multi-bot capability for their Poe platform, we're excited they're validating the approach we pioneered nearly a year ago. As Quora CEO astutely points out, different AI models have unique strengths, and the ability to easily leverage and combine them is key to unlocking optimal workflows.
Dust's multi-assistant feature provides an even stronger capability by enabling users to query, compare seamlessly, and chain together multiple assistants in a single thread with the company context.
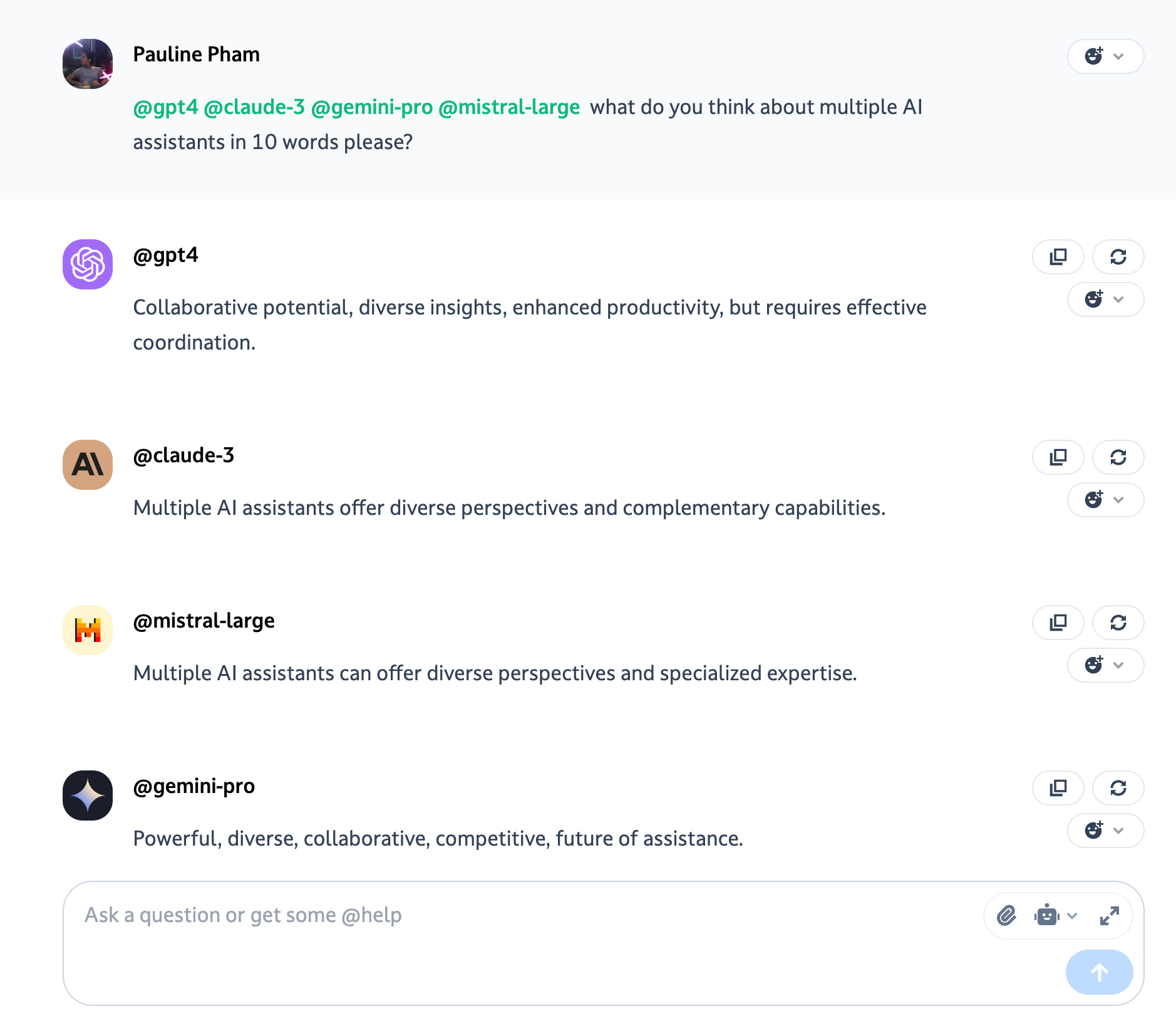
Why multiple assistants? A few key reasons
Put simply, Dust multi-assistants allow you to chat with multiple custom assistants powered by different LLMs or a different set of instructions in a single conversation thread. Different LLMs have different strengths, weaknesses, and areas of knowledge.
Multi-assistant workflows help users find the best combination of assistants for each work step by enabling easy comparison of outputs from various models, instruction sets, and data sources and the ability to summon specialized assistants created with the company's data with a simple @-mention.
- Custom assistants with access to selected company data outperform generalist models for specific tasks. An assistant created with your code documentation and codebase to help your engineering team write better code will give better results than a general model when reviewing a pull request. An assistant using your company's customer support history will handle tickets better than a generic chatbot.
- You can chain assistants. For example, you might start by querying a model assistant to learn about a topic or a custom assistant that has access to curated knowledge and then call on a writing-focused assistant to turn retrieved information into a polished output to share with your team.
- You can empirically test what works best for your needs. With Dust, you can easily compare outputs from Claude, GPT-4o, Gemini, and Mistral Large on the same task to see which gives the best results. Mix and match until you find your winning combo.
- It future-proofs your setup as new models emerge. As transformative new AI capabilities arise (like our current work to turn assistants into agents), you can seamlessly incorporate them into your workflows without rebuilding everything around a single system.
Making LLMs accessible and intuitive for every employee.
Our early development of the multi-assistants feature was not merely a technological achievement but a commitment to ease everyone's use of LLMs without the help of an engineer. As outlined in Stanislas Polu's foundational article on the motivations behind Dust, our approach has always been about identifying and unlocking product-layer barriers to AI adoption in team settings. This foresight has allowed us to participate in the evolution of generative AI and actively shape it in ways that are most beneficial to our users.
Giving employees the power to create their AI assistants has been core to our vision. We don't believe in a top-down, one-size-fits-all approach to workplace AI. The people closest to the work know their needs best. With a multi-assistant platform, they can experiment to find the optimal combination of models and data sources for their specific context.
Unlike narrow, siloed vertical solutions, Dust assistants can operate horizontally across all the applications and systems that modern work touches. This allows them to automate and supercharge cross-functional workflows, which are organizations' lifeblood.
Critically, though, humans remain at the helm. Dust is not pursuing automation for automation's sake but amplifying the human factor - individuals' and teams' judgment, creativity, and domain expertise.
We need to shift our mindset to harness the power of LLMs truly. These tools are not meant to replace human intelligence but to augment it. Effectively prompting an LLM requires clearly articulating your context and objectives - a skill that is developed through hands-on experience and experimentation. At Dust, we aim to facilitate this learning process at scale, making LLMs accessible and intuitive for every employee. We believe the transformative potential of LLMs lies in the underlying technology and the human capacity to wield it.
With Dust, each person can summon a unique combination of AI capabilities that unlock their potential. The future belongs to empowered employees equipped with a collection of specialized AI assistants and, very soon, agents that evolve with the state of the art.
We're excited to be at the forefront of this shift and can't wait to see the workflows our customers build as they realize the potential of multi-assistant/agent collaboration.