Happy Acceleration in 2024!
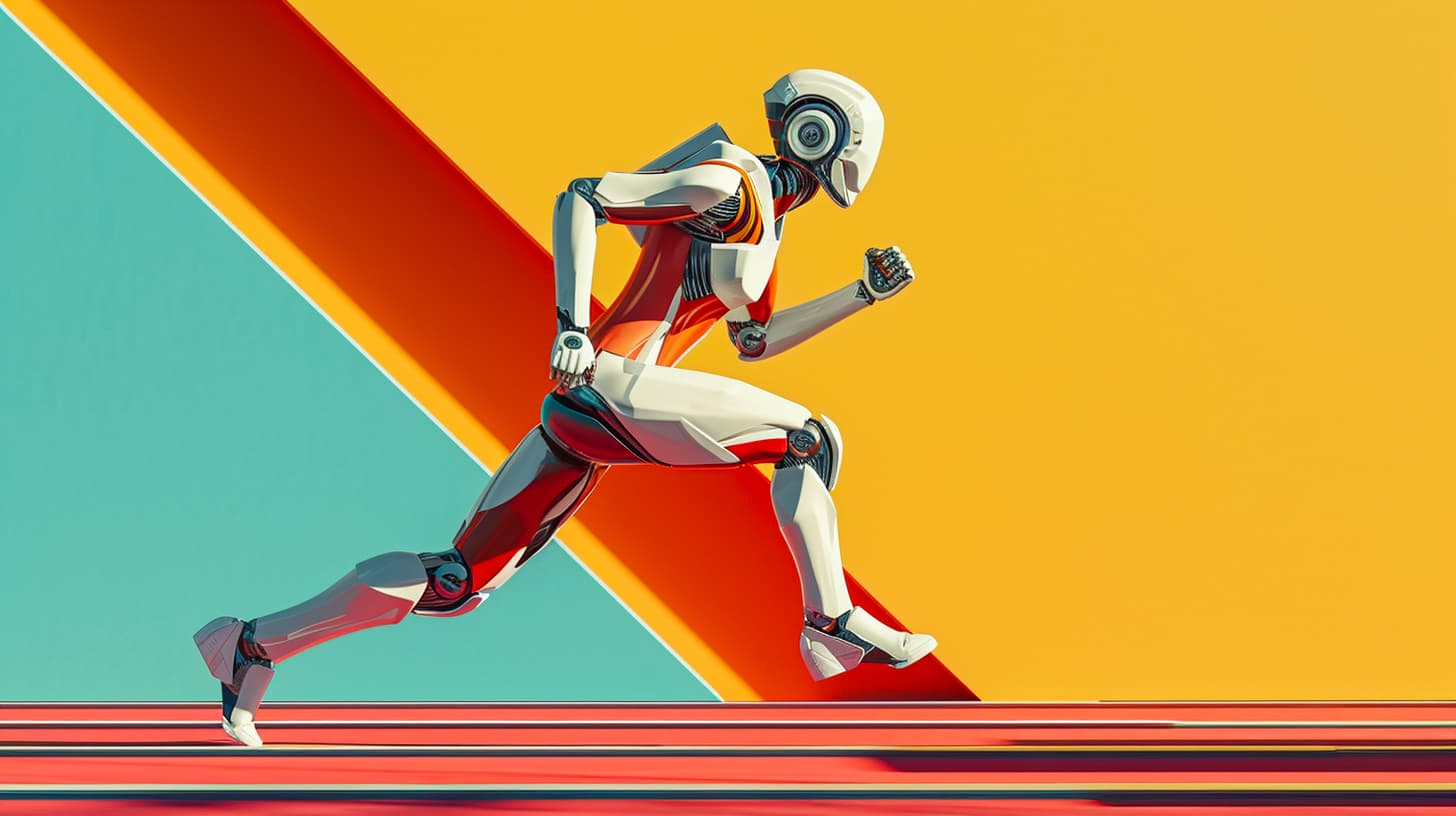
2023 was a year of exciting exploration for many teams as they sought to understand the capabilities of generative AI (“GenAI”) and the impact it could have on what they sell but also on how they work to sell it.
In 2024, we expect many companies to decide on a set of solutions to accelerate internal collaboration and information management: searching and processing information, automating large chunks of manual workflows, etc. 2023 has been the year of talking about leverage from GenAI, and many want 2024 to be the year of rolling it out at scale.
With the experience we’ve accumulated from helping thousands of users across fast-moving implement solutions that work for them, we thought we’d share a few observations and beliefs we’re holding more strongly than ever as we enter 2024.
Acceleration from Experimentation
GenAI is moving very quickly. We’ve found that teams that embrace experimentation early and broadly tend to find deeper and sometimes unsuspected pockets of value across functions and across workflows. This requires sufficient education on how LLMs work: models’ quirks, prompting, and of course operating with retrieval-augmented generation—generating content with the context of information that’s been previously retrieved. The earlier you start, the earlier you learn, and it’s become clear that having an isolate team test solutions with limited feedback from other functions can slow that learning process down dramatically. It can be tough to predict which teams and individuals will actually be comfortable experimenting.
As anecdotal examples:
- After 30 days, daily active usage of GenAI-powered agents to complete tasks in one company that adopted Dust and promoted early experimentation internally was an order of magnitude higher than in another similarly-sized team that had decided on a limited pilot within the customer service department.
- Within 3 weeks, both usage and satisfaction increased 4-fold after a different lead was assigned to the experimentation of internal information retrieval agents at one company. This second lead didn’t have more experience with LLMs and hadn’t initially been thought of, but they demonstrated a starkly different attitude towards trying new things, asking questions, and relaying lessons learnt to their teammates.
- Agents with the highest usage at one company came from a division that hadn’t initially been included in the discussions around the adoption of Dust. The talent team decided to experiment with agents to help hiring managers better navigate the numerous policies for this fast-growing team of 1200 people and has since doubled down based on early results.
Acceleration from Openness
With its unique ability to recombine information from various sources, GenAI proves most useful when users aren’t quite sure where the right sources are in the first place.This does greatly favour teams that give broader access to information internally, avoiding the hurdles of access and permission management on data that wasn’t really that sensitive in the first place but became siloed as a result of passive or misaligned decisions on internal transparency.
As examples:
- At Alan, a 550-person team using Dust, the deeply-held culture of radical internal transparency has allowed experimentation across teams with limited friction on the availability of data sources to design and distribute complex workflow agents serving audiences across functions.
- At another 400-person company using Dust, agents generating information on past Slack discussions to help avoid repetitive questions on poorly documented or sometimes subtle use cases weren’t able to perform as effectively because of segregation of important sources of information between product and customer service teams “as a default”. Fixing this proved crucial.
Acceleration from Customisation
Those actively using foundation models have experienced the limitations of agents that are too general and don’t quite deliver the level of performance required to truly accelerate teams on demanding tasks. Tone of voice, types of results to exclude, format of the output… All these parameters are tiresome to “prompt” at each time a user starts a new conversation or triggers a new workflow. In November, Open AI confirmed wanting to support users with GPTs that could support saving some of these preferences.
An important bet Dust made in August 2023 was to make it easier to create, distribute, and maintain custom agents that perform tasks based on dedicated specifications and instructions. It wasn’t obvious that having more agents would help: there was a risk of confusion among team members. A few months later, teams that have used Dust to develop team- and sometimes task-specific agents aren’t looking back. Having a robust platform to support personal, team-level and company-level agents has helped a lot here and we’re excited to provide continued support to this in 2024.
As examples:
- Most members at some of Dust’s most active customers worry “little or not at all” about having access to a large number of agents, as long as the descriptions of their behaviour and specs and their authors are easy to access. In some cases, they’ve also appreciated being able to duplicate agents to further customize them to their own, specific needs.
- Some teams on Dust now have 1 custom agent to every 3 or 4 members on their team! This isn't broadly the case yet, but we believe it’s the direction we’re headed in and we’re excitedly observing that activity on Dust correlates positively with the number of custom agents a workspace has.
- This author personally uses highly custom agents to prep weekly meetings: @weeklyshipped, @weeklyincidents and @weeklyfeedback generate nicely formatted tables that easily populate the team’s pre-read document.
Acceleration… THE role of 2024?
Most companies have set up an “AI task force” in 2023 to assess the potential, threats and opportunities of GenAI technology. Executive sponsorship and a close connection with a business-minded IT/security teams are strong predictors of success for these task forces.
But there’s also signs that the most powerful members of these teams are combining some skills to take on a new role we’re tempted to describe as “acceleration operations”: bringing together a technical understanding of LLMs and the ability to drive teams to make the appropriate internal data sources available, identifying use cases, rolling out experimental agents quickly to get teams going, and continuously improving them based on observed performance across teams, new models, etc...
Hey, if 2024 is the year of GenAI going into production at scale for internal collaboration, we believe it might be the year of acceleration ops.
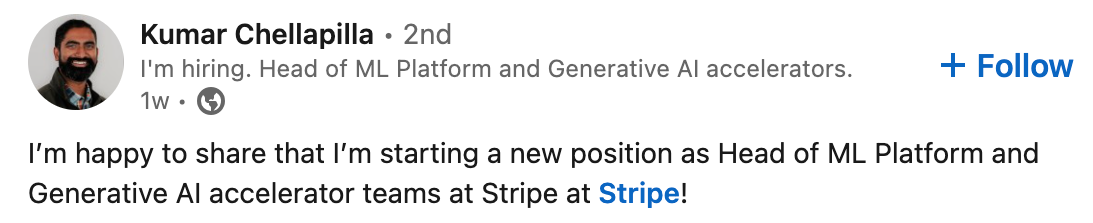
Based on our observation of the leading traits the most effective team members among Dust’s customer demonstrate, here’s a job description we believe more and more teams will publish some version of in the weeks and months to come:
What you’ll do
You’ll empower teams to best leverage GenAI technology, distributed internally via a platform like Dust, to give them access to the best LLMs and most advanced toolkit to work better and faster together.
Who you are
We’re looking for someone with the minimum requirements below. Preferred qualifications are a bonus. If you don’t meet all the requirements but have a high degree of confidence in your fit for this new role, we’d encourage you to apply and would love you to tell us more about why you strongly believe you’re a fit.
Minimum requirements
- Comfortable in a fast-paced environment, and with technology that is evolving rapidly (think: big news every week or every few weeks).
- Genuine enthusiasm about the potential for LLMs to change the nature of the day-to-day of knowledge workers.
- Direct experience with LLMs: testing them, prompting them, using them in the context of achieving tasks. You know what temperature, context window, function calling, retrieval, embedding, and code interpretation mean in the context of LLMs.
- Experience with rapid prototyping, experimentation, and iteration.
- Excellent cross-functional communication and collaboration skills.
- Ability to identify the correct metrics to incentivise and measure impact.
- Strong intuition on the right balance to strike between good and perfect.
- Good understanding of data models when discussing requirements with engineering teams or SaaS providers.
Preferred qualifications
- Experience as a project manager, product manager, product operations
- Experience working with data sensitive applications
- Experience with scripting languages
Best speed and leverage for the New Year!
We’re convinced of the radical potential of LLMs to accelerate fast-moving companies with ambition. We’re already observing how Dust’s customers are deploying agents across teams to enhance internal collaboration and handle heavy or repetitive workloads. We’re betting on leverage with GenAI being a focus for the most forward-looking teams, and on leverage operations being a promising opportunity for many driven individuals working in them.
Best speed to them all, and let us know if Dust can help!